Our services
ION Data Science offers a variety of services to help businesses and organizations leverage data and analytics to make informed decisions and drive growth. Here are some of the services offered by ION Data Science:
Nuestros servicios incluyen
Regression (value estimation)
Class probability estimation (classification)
Similarity matching
Clustering
Co-occurrence grouping
Profiling (behavior description)
Link prediction
Data reduction
Causal modeling
Regression (value estimation)
For each individual, it predicts /estimates the value of some variable belonging to that individual. For example, the typical question to solve with regression could be: “How much will the “customer x” use this service?” In this case, the property (variable) to be predicted is “service usage”. Then, by looking their historical usage of other individuals in the population a model to predict this variable (“service usage”) should be generated. It means, given an individual, a regression estimates a particular variable of that individual.
What is the difference between regression and classification? Basically, classification estimates if something will happen, whereas regression estimates how much it will happen.
Class probability estimation (classification)
It predicts for which of a (tiny) set of classes an individual belongs to. Usually, these classes are mutually exclusive. For example, the typical question to solve with classification is: “Among all the customers of A-corp, which are likely to respond to a given specific offer?” Then, the two classes could be called: “will respond” VS “will not-respond” costumers. Then, given a new individual “customer x”, data mining procedure will produce a model that determines which class that “customer x” belongs to.
A class probability estimation (“scoring”) is applied to represent the probability (or other quantification of likelihood) that the given “customer” belongs to each class (in this case, the probability that “customer x” belongs to “will respond” class and the probability to belongs to “will not-respond” class).
Similarity matching
It identifies similar individuals based on data known about them. It can be used directly to find similar entities. One example, “A-corp“ wants to find other companies similar to their best companies customers. Then, “A-corp” could focus their sales force on these best opportunities. We can use similarity matching based on what we call “firmographic” data that describes the characteristics of these companies.
Or another example of this technic is when make product recommendations are given to you in many seller websites. Similarity matching measures underlie certain solutions to other tasks such as regression, classification or clustering.
Clustering
Not driven by any specific purpose, this task groups individuals (in a population) together by their similarity. For example, the typical question to solve with clustering could be: “Do our customers form natural segments or groups?”. In preliminary domain exploration, this technique could be crucial to see which natural groups exist because these groups detected may suggest other data-mining approaches.
Another typical question to solve with clustering is: “How should our sales teams be structured (based on our customer care)?“ or “What products should we develop (based on our customer care)?”. It means that clustering could also be used as input to decision-making processes.
Co-occurrence grouping
> Association rule discovery/frequent item-set mining/ market-basket analysis.
It finds associations between entities based on transactions involving them. For example, the typical question to solve with co-occurrence grouping could be: “What products are usually purchased together?” Clustering task looked at similarity between items based on “their attributes”, whereas co-occurrence grouping considers similarity of items based on “their appearing together in transactions”.
Let me explain you why it is also typically called as market-basket analysis. For example, this technique could be used to analyze purchases from a supermarket and uncover that “product A” is purchased together with “product B” much more frequently than marketers might expect. Then, decisions could be taken upon this discovery such as a combination offer, product display or a promotion. It could be used to associate pairs of products frequently purchased by the same people.
The result of this task is a description of items that occur together that usually include statistics on the frequency of the co-occurrence and the estimation of how surprising it is.
Profiling (behavior description)
It characterizes the typical behavior of a population, group or individual. The typical question to solve with profiling could be: “What is the typical computer usage of this customer segment?” People have not simple behavior description. Profiling computer usage could require a complex description of weekend and working days night usage averages, typing minutes, etc.
Usually, it is interesting to decompose behavior in groups of users or even individuals but it could be done for the entire population. This technique is often used to detect anomaly applications such as Internet illegal intrusions or fraud detection. For example, if we know how a person typically uses a web service, we can determine whether a change in that behavior fits that profile or not. Then, we use this mismatch level as a suspicion score and if, it is large enough, take appropriate measures.
Link prediction
It predicts connections between data items. Link prediction shows the existence of this link and it estimates the strength of this link. The typical question to solve with link prediction could be: In social networks, “Since you and Mike share 34 friends, maybe you would like to be friend of Mike?”. And, it also estimates the strength of the link.
Following the example, if you share with Mike and Tyson the same number of friends but the friends that you share with Mike are living in the same area than you but Tyson’s friends not, it could be determined that your link with Mike is stronger than your link with Tyson.
Data reduction
It takes the important information of a large set of data to convert it in a smaller set of data that contains much of the most important information. Then, it is easier to deal or to process with the smaller dataset. This seems trivial but actually the smaller dataset usually reveal better information. The typical question to solve with link data reduction could be: “Observing our massive dataset of consumer preferences, could we reveal information about consumer preferences (for example, genre preferences)? This technique usually involves loss of information.
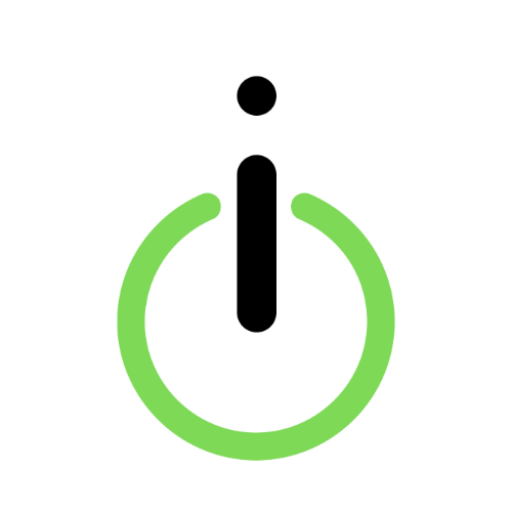
Causal modeling
It helps us understand what actions (or events) actually influence others. One example, imagine we use a predictive model to target advertisements to consumers, and we observe that this measurement produce purchase behavior changes. Is this because the advertisements influence effectively the consumers? Or is this because our predictive model simply works well identifying those consumers who would have purchased even if the advertisement campaign has not launched? Then, we use controlled experiments (such as A/B tests) or sophisticated methods for find causal conclusions between observations. Casual modeling is a “counter-factual” analysis. It means, we want to understand what would be the difference between two (or more) situations (which theoretically cannot given at the same time) where the given event is “applied” or “not applied”.
This experimentation could be too expensive due to the large number of data required. The business needs to decide what investment decides to apply to get the enough level of confidence to be able to conclude the assumptions. Furthermore, in many cases, assumptions could make causal conclusions invalid. For example, the “placebo effect” could appear. We should carefully design randomized experimentation in this case of applications. Always, a careful data scientist should include with a causal conclusion the exact assumptions must be made in order to hold the causal conclusion.